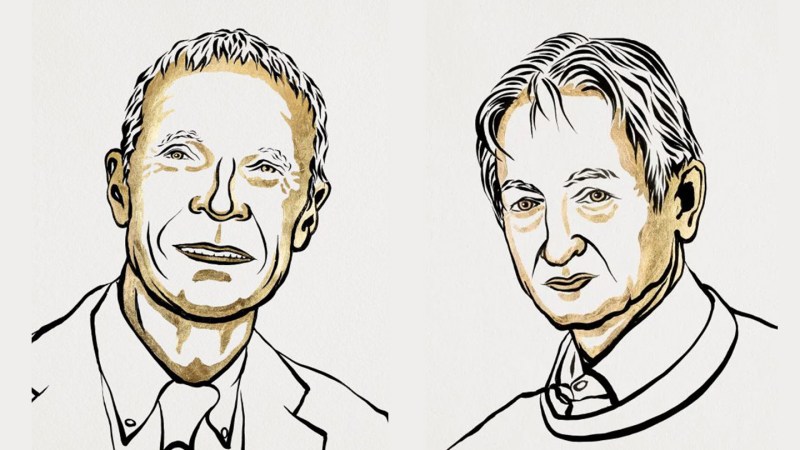
As artificial intelligence explodes in popularity, two of its pioneers have been awarded the 2024 Nobel Prize in Physics.
The award goes to John Hopfield and Geoffrey Hinton “for fundamental discoveries and inventions enabling machine learning with artificial neural networks,” the Royal Swedish Academy of Sciences in Stockholm announced on Oct. 8. These computational tools, which aim to mimic the workings of the human brain, underpin technologies such as image recognition algorithms, large language models including ChatGPT, soccer playing robots and more (SN: 2/1/24; SN: 24.5.24).
The award surprised many people, as these developments are usually related to computer science rather than physics. But the Nobel committee noted that the techniques were based on the methods of physics.
However, no one was more shocked than Hinton himself: “I’m shocked. I had no idea this would happen. I am very surprised,” he said by phone during the press conference.
The techniques have supported a number of scientific advances. Neural networks have helped physicists deal with large amounts of complex data, allowing important advances that include imaging black holes and creating materials for new technologies such as advanced batteries.SN: 4/13/23; SN: 16.1.24). Machine learning has also made advances in the biological and medical fields, with the promise of improving medical imaging and understanding protein folding (SN: 17.6.24; SN: 23.9.23).
“This award confirms the fact that AI is not just a niche technology – it is a scientific revolution with interdisciplinary impact,” says AI researcher Craig Ramlal of the University of the West Indies in St. Augustine in Trinidad. “More importantly, this award legitimizes AI as a tool for understanding and simulating the natural world, which we hope will drive more innovation.”
Neural networks are designed to identify patterns in data, rather than doing explicitly programmed calculations. They are based on a network of individual elements called nodes that are inspired by individual neurons in the brain. Training the neural network by feeding it data improves its ability to make accurate inferences by optimizing the strength of connections between nodes.
In 1982, Hopfield, of Princeton University, created an early type of neural network, called a Hopfield network, that could store and reconstruct patterns in data. The network was similar to magnetic materials in physics, in which atoms have small magnetic fields that can point up or down, similar to the values 0 or 1 at each node of a Hopfield network. For any given configuration of atoms in a material, scientists can determine its energy. A Hopfield network, after being trained on a set of patterns, minimizes an analog energy to discover which of these patterns is hidden in the input data.
“In my opinion, physics is trying to understand how systems work. Systems are made of parts. These parts interact,” Hopfield said in remarks delivered virtually during a news conference in Princeton.
Hinton, of the University of Toronto, built on that technique, creating a neural network called a Boltzmann machine, which is based on statistical physics, including the work of the 19th-century Austrian physicist Ludwig Boltzmann. Boltzmann machines contain additional nodes that are hidden – they process data but do not directly receive input. Different possible states of the model have a different probability of occurring. These probabilities are determined by the Boltzmann distribution, which describes the configuration of many particles, such as molecules in a gas.
“The work that Hopfield and Hinton did has just been transformative, not only for the research communities developing AI and neural networks, but also for many aspects of society,” says computer scientist Rebecca Willett of the University of Chicago.
The two winners will share the prize of 11 million Swedish kronor, or about $1 million.
“I was glad to hear that, actually. It was a massive surprise,” says AI researcher Max Welling from the University of Amsterdam. “It has a very clear connection to physics… The models themselves are deeply inspired by physics models.” Moreover, the discovery made many developments in physics possible, he says. “Try to find a technology that has had a bigger impact on physics, especially on the methods side. It’s difficult.”
Since the 1980s, researchers have greatly improved these models and scaled them up dramatically. Deep machine learning models now have many layers of hidden nodes and can boast hundreds of billions of connections between nodes. Huge amounts of data are used to train networks, scraping the internet for text or images to feed into them.
While artificial intelligence technologies based on neural networks are capable of achievements unimaginable in the 1980s, the technologies still have a host of pitfalls. Many researchers now focus on understanding how the prevalence of machine learning can have negative impacts on society, such as reinforcing racial prejudice, facilitating the spread of misinformation, and making plagiarism and fraud quick and easy (SN: 9/10/24; SN: 2/1/24; SN: 4/12/23).
While some scientists, including Hinton, worry that AI could become superintelligent, the way AI is trained differs from human learning models, and many researchers disagree that AI is on a path to world domination (SN: 28/2/24). Artificial intelligence models are famous for ridiculous mistakes that defy common sense. Scientists are still actively working to determine how terms like “understanding” can be applied to machine learning systems and how best to test their abilities (SN: 7/10/24).
“There are real concerns about how [AI is] it will affect work and the labor market, as it enables misinformation and data manipulation,” says Willett. “I think these are very real concerns here and now. That’s because people can take these tools and use them for malicious purposes.”
#Discovery #key #machine #learning #tools #wins #Nobel #Prize #Physics
Image Source : www.sciencenews.org